
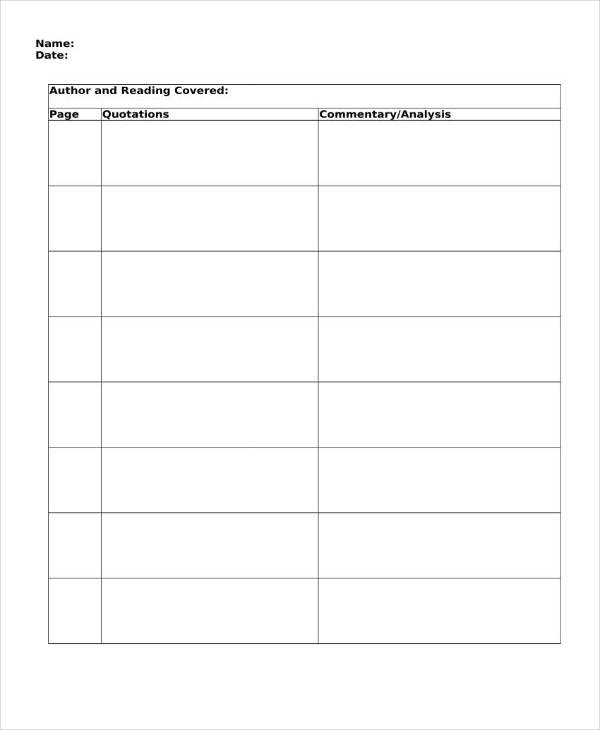
The implementation of in silico models based on FE techniques can be useful for accelerating the development of recyclable CFRTPs for large-scale production, allowing significant weight reduction and lower greenhouse emission without diminishing their reliability and safety throughout their useful life. In addition, this model was used to further optimize the component geometry.
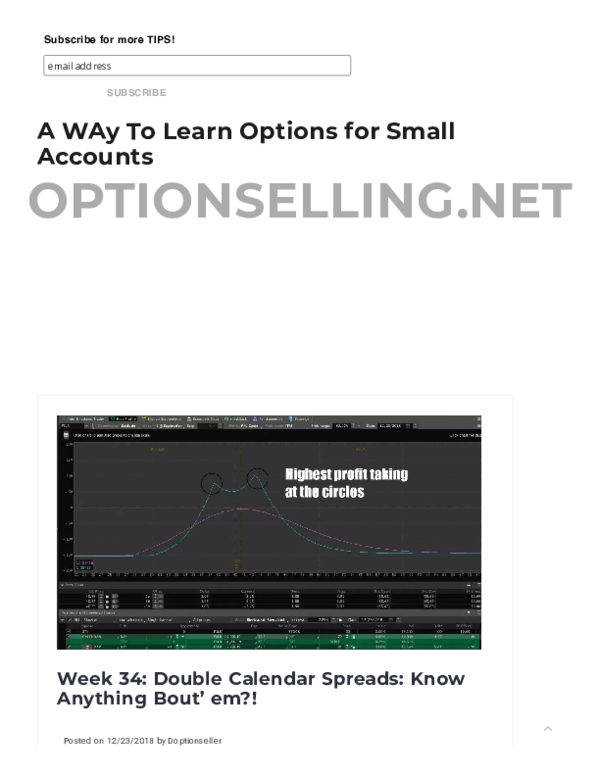
The obtained results were fitted with the test results to validate the developed FE model. This method was based on a Halpin-Tsai model and classical lamination theory with a 3D expansion for out-of-plane mechanical property calculation, wherein Tsai's modulus was obtained for checking purposes. Furthermore, a complete mathematical procedure was implemented to address the lack of information on the mechanical moduli and Poisson's ratio in the material datasheet for the FE simulation. The experimental results were used to validate those obtained using a finite element (FE) model based on the fabricated geometry and mechanical properties of the CFRTPs laminate. This was achieved by developing ad hoc tooling for axial testing. In this study, the mechanical behavior of a CFRTPs top mount damping component made of polyamide 6 (PA6) reinforced with long glass fibers (47% in volume) and installed in an automobile differential system was evaluated. Geometrical optimization is typically based on trial-and-error processes, and delays due to the manufacturing of different prototypes increases the development cost of the final product. The manufacturing of thermoformed components of continuous fiber-reinforced thermoplastics (CFRTPs) relies on an optimization process owing to a demand for quality and the mechanical requirements. This machine learning methodology can be a starting point for more sophisticated simulation models, such as thermo-mechanical loads, complex structures, other composite families, and above all it can simplify the optimization process of composite structures. The results show that ridge regression can make predictions with the highest accuracy of up to 99% and is faster than simulations by three orders of magnitude, also allowing us to efficiently search for the best Double-Double angles.
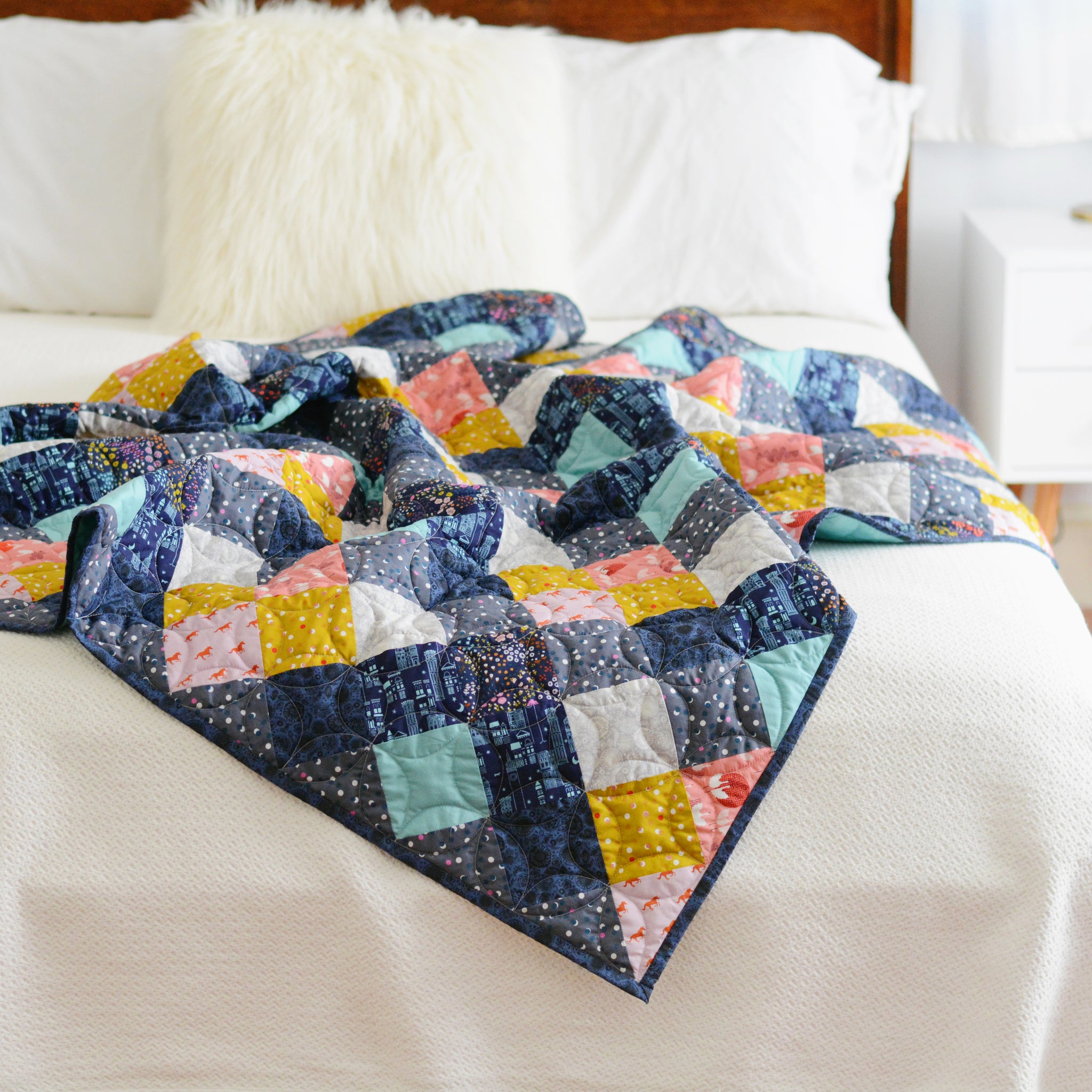
Multiple machine learning methods are applied to predict the displacement field of a 2D wing-shaped Double-Double composite model under three loading conditions (tension, shear and bending). Here we propose to accelerate the simulation process of composite structures by making predictions using a data-driven strategy and adopting a novel family of composite laminates, named Double-Double, which homogenizes the stacking sequence with fewer plies and only two ply-angles. Furthermore, numerical simulations, such as finite element method, include large computational costs that often push cost-benefit compromises. Nevertheless, these processes usually involve many variables derived from traditional legacy QUAD laminates. Current composite design processes go through expensive numerical simulations that can quantitatively describe the detailed complex stress state embedded in the laminate structure.
